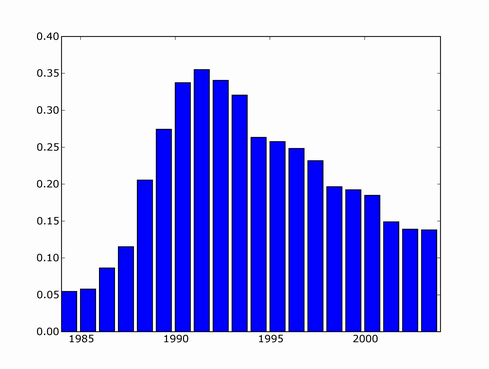
Since then, there's been several major NN developments, involving deep learning and probabilistically founded versions so I decided to update the trend. I couldn't find a copy of scholar scraper script anymore, luckily Konstantin Tretjakov has maintained a working version and reran the query for me.
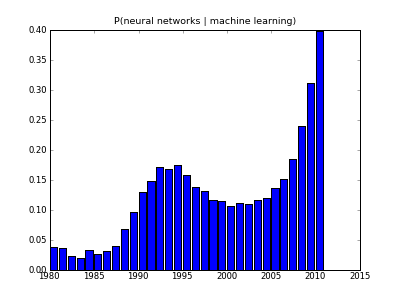
It looks like downward trend in 2000's was misleading because not all papers from that period have made it into index yet, and the actual recent trend is exponential growth!
One example of this "third wave" of Neural Network research is unsupervised feature learning. Here's what you get if you train a sparse auto-encoder on some natural scene images
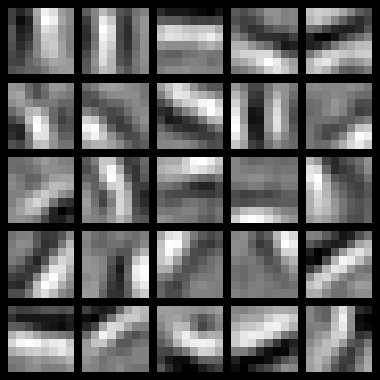
What you get is pretty much a set of Gabor filters, but the cool thing is that you get them from your neural network rather than image processing expert
155 comments:
"Don't call it a come-back"
http://en.wikipedia.org/wiki/Mama_Said_Knock_You_Out_%28song%29
Maybe you should mention a reference to Hintons 2006 work and how it affects all deep learning architectures?
I would if I knew, what's so great about Hinton's 2006 paper?
Hinton's "reduced Boltzmann machine" is a deep neural network -- many layers. For some reason, a lot of the old NN literature only considered one or two level deep NNs. More layers = better.
I would recommend his tech talk:
http://www.youtube.com/watch?v=AyzOUbkUf3M
The problem is that backprop can't efficiently learn multilayer networks to result in deep architecture. Network can have 10 layers but it's still shallow. So recent advances like RBMs or sparce autoencoders making this "come back".
As far as I understood, Hinton's 2006 paper was what started the whole autoencoder and pretraining wave...
Maybe it's a question that ought to be asked to either:
- the stats or metaoptimize Q&A
- Andrew
According to the wikipedia, the auto-encoders algorithm seem to help speed up the back propagation step (http://en.wikipedia.org/wiki/Auto-encoder ).
I am curious too.
Cheers,
Igor.
What a fantastic read on Hadoop.This has helped me understand a lot in Hadoop course. Please keep sharing similar write ups on Hadoop.Guys if you are keen to knw more on Hadoop, must check this wonderful Hadoop tutorial and i'm sure you will enjoy learning on Hadoop training.https://www.youtube.com/watch?v=ApR6pHN-m6M
This neural networking side should have been there so that the overall factors lying to this could be into our mind. https://www.academicposter.net/faq-how-to-make-an-academic-poster/ to see more about the writing tips.
Who would ever thought that the Mary Wood will go this long. This is really some lovely news about her that she can do better in the acting profession in her way. https://radiology.residencypersonalstatements.net/radiology-personal-statement-writing/ & it will give you some amazing idea and very effective for the papers writing.
It is really a good decision to know about the register number in really easy way which let the people in relief that there had no any tough situation will face in later time. https://www.writemyessays.org/how-to-write-a-3-page-essay-fast/ for the students that is very helpful for the writing services.
Excellent blog.
Machine Learning Training course
Great post. I absolutely love to find unique places like this. It really looks super creepy though!! Best Machine Learning Training in Chennai | best machine learning institute in chennai | Machine Learning course in chennai
there has so many reason behind the love of this philosophy subject as mentioned http://www.applicationessayprompts.com/answers-to-college-essay-prompts/help-with-writing-a-college-application-essay/
/ as it gives you that chance to know about people's mind.
If they are really come back to the exact point http://www.conferenceresearchpaper.com/word-to-speech-converter/ and situation then none could overtake them like they was in those past time history. Check about them in here.
Thank you .its a nice blog.
click here:
Data Science Online Traning
Very nice to read keep on posting
Machine learning training in chennai
very nice article Leadingmachine learning training in ameerpet
Informative post for machine learning
data science training institute in chennai
It's really a nice experience to read your post. Thank you for sharing this useful information
big data hadoop training cost in chennai | hadoop training in Chennai | best bigdata hadoop training in chennai | best hadoop certification in Chennai
THANKS FOR THE INFORMATION....
Digital Marketing Internship Program in BangaloreDigital Marketing Internship Program in Bangalore
Good Post. I like your blog. Thanks for Sharing
Machine Learning Training in Noida
Great Article
Final Year Project Domains for CSE
Final Year Project Centers in Chennai
JavaScript Training in Chennai
JavaScript Training in Chennai
Thanks for sharing such a great blog Keep posting..
Machine Learning Training in Delhi
Nice Blog..thanks for sharing this information..
Best Data Science Training in Chennai
Top 5 Data Science Training in Chennai
Data Science training Course Content
Data Science Training in Velachery
Data Science Training in omr
Data Science Training in vadapalani
Data Science Training in Chennai
Data Science Courses in Chennai
Data Science Training Institute in Chennai
Data Science online course
Data Science with python training in chennai
Fleet Maintenance software
Money Gadget
Money Gadget
Money Gadget
Money Gadget
Money Gadget
Money Gadget
Money Gadget
Money Gadget
Money Gadget
Money Gadget
Money Gadget
Money Gadget
Money Gadget
Money Gadget
Money Gadget
Money Gadget
Shield Security Solutions Offers Security Guard License Training across the province of Ontario. Get Started Today!
Security Guard License | Security License | Ontario Security license | Security License Ontario | Ontario Security Guard License | Security Guard License Ontario
Aivivu chuyên vé máy bay, tham khảo
giá vé máy bay tết 2021 vietjet
vé máy bay đi Mỹ giá rẻ
ve may bay di Phap gia bao nhieu
giá 1 vé máy bay đi hàn quốc
vé máy bay vn airline đi nhật
từ Việt Nam bay sang Anh mất bao lâu
mua vé máy bay giá rẻ nhất
It is great post! I think you can make youtube channel about learning machines. People don't know much about it, but it is very interesting. So if you create it you will need this site https://soclikes.com/ to get youtube likes and views
Săn vé máy bay tại Aivivu, tham khảo
vé máy bay đi Mỹ bao nhiêu
chuyến bay từ mỹ về việt nam tháng 1/2021
chuyến bay nhật bản về việt nam
giá vé máy bay từ Vancouver về việt nam
It was amazing and so helpful. Thank you.
classified ads platform in Bangladesh
classified sites in Dhaka
Ertugrul game play free in mobile
Wow, incredible weblog layout! How long have you ever been running a blog for? you made running a blog glance easy. The full look of your website is excellent, as well as the content.cosmetic packaging boxes wholesale | cosmetic packaging boxes wholesale
the data analysis tool SSAS which is used to make analyze and parse all that data so that business value can be derived from it. It is an online analytical processing tool that helps to work with streaming data and analyze it to help businesses gain competitive advantage. MSBI Training
Nowadays, the world has become a small place because of the internet. Whatever information we need, we go to a search engine to search for that.
Great post I like it very much keep up the good work.
packaging for Corrugated
Cookie box USA
The importance of self-service tickets for healthcare administrators
Health center administrators are the connecting link between patients and doctors. From scheduling appointments to managing transactions, their database needs to be constantly updated.
Answering repetitive questions
Administrators often have to deal with repetitive questions that emerge around specific purpose forms, doctors' specialties, accepted insurance companies, and many more. Using modern service desks allows them to provide automated answers to specific questions as they are asked.
Ensure data security
Many health centers are required to comply with specific data compliance regulations to keep their patient records safe. They cannot allow unauthorized personnel to disclose or access sensitive data.
Modern service desks allow healthcare administrators to keep all data sets, including documents, files, medical records, and patient reports, within one secure platform. It enables medical institutions to store paperless documents in a multi-layered security system and eliminate a variety of potential threats.
The last word
Looking at the functions of a modern service desk and its self-service ticketing system in the healthcare sector, it can be said that such a digital approach is the future of the sector. It is recommended that healthcare institutions switch to automated platforms to take advantage of the care services provided to their patients, doctors, and administration.
Share this post: Ai service desk | Conversational AI service desk
Fancy name plates are a great way to personalize your new home. These Fancy Name Plates for Home are easy to install, and look elegant in any entrance or hallway. With choices ranging from wood plaques to granite plaques, you can choose the right fancy name plate for your home!
Good content. Clear explanation. Big thumbs up for this blog. Keep sharing some more blogs again soon. Thank you.
Machine Learning Training in Hyderabad
hi
Yay google is my queen assisted me to find this great web site! 카지노사이트
카지노사이트 Hi there, I found your blog by the use of Google while looking for a related subject,
your site came up, it appears good. I've bookmarked it in my google bookmarks.
토토 I have been browsing online greater than three hours lately, yet I by no means discovered any interesting article like yours. It is pretty price sufficient for me. In my opinion, if all website owners and bloggers made good content material as you probably did, the internet will be a lot more useful than ever before.
토토사이트 Nice post! This is a very nice blog that I will definitively come back to more times this year! Thanks for informative post
"Hi my loved one! I wish to say that this post is amazing, nice written and come with almost all vital info's. I would like to peer extra posts like this"
무료야설
We are linking to this particularly great content on our site. Keep up the great writing. Pretty valuable material, overall I consider this is worth a bookmark, 룰렛
Wonderful web site. Plenty of useful info here. I am sending it
to several pals ans additionally sharing . 바카라사이트
This is my first visit to your blog! We are a team of volunteers and starting a new initiative in a community in the same niche. Your blog provided us beneficial information to work on. 카지노사이트프로
Some genuinely interesting points you have written. Helped me a lot, just what I was looking for Very clean and excellent user friendly style and design, as well as the content. You’re an expert in this topic! 스포츠토토
I really appreciate this post. I’ve been looking all over for this! Thank goodness I found it on Bing. You’ve made my day! Thank you again 바카라사이트
Hi, just required you to know I he added your site to my Google bookmarks due to your layout. I've came across. It extremely helps make reading your blog significantly easier. 카지노사이트
You actually explained this terrifically!
Oh my goodness! Awesome article dude! Thanks, Numerous tips!
Try to check my webpage :: 경마
I am overwhelmed by your post with such a nice topic. Usually I visit your site and get updated through the information you include but today’s blog would be the most appreciable. 메이저사이트
What an interesting article! I'm glad i finally found what i was looking for. 경마
This blog iswhat im exactly looking for. Great! and Thanks to you. 토토사이트
Your work is very important to me so I liked it. If you like pets you can see here Wild Animals.
토토 This design is wicked! You obviously know how to keep a reader
entertained. Between your wit and your videos, I was almost moved to start my own blog
(well, almost…HaHa!) Wonderful job. I really loved what you had to say, and more than that, how you
presented it. Too cool!
스포츠토토 Wonderful work! This is the kind of information that are meant to be shared around the net.
Disgrace on the search engines for not positioning this put up higher!
Come on over and discuss with my site . Thanks =)
카지노사이트 I have been surfing on-line greater than three hours lately, but I never discovered any attention-grabbing article like yours.
It's lovely price sufficient for me. Personally, if all web owners and bloggers made excellent content material as
you did, the web will be a lot more useful than ever before.
바카라사이트 I think the admin of this site is really working hard for his site, for the reason that here every information is quality based
information.
Well, You know what , You deserve the high amount of appreciation for this work and I must say that you are a top notch information breaker. Online Shopping in Pakistan
Well I highly appreciate the amount of time you have put in writing this content down. Love this Best barrier door mats
Great post. I absolutely love to find unique places like this. It really looks super creepy though!
Alignment near me
roadside assistance services
Trailer repair
Trailer repair shop
Truck towing near me
mobile truck repair
Nice post. Thanks for sharing! I want people to know just how good this information is in your article.
Microblading Near Me
Permanent Makeup Near Me
Microneedling Near Me
Cryotherapy Near Me
Microdermabrasion Near Me
Waxing Near Me
Thank you for posting such a great article! It contains wonderful and helpful posts. Keep up the good work
Har Ghar Nal Yojana 2021
fantastic publish, very informative. I wonder why the opposite experts of this sector do not understand this. You should continue your writing. I’m sure, you’ve a great readers’ base already!
https://www.safecasinosite.net
While looking for articles on these topics, I came across this article on the site here. As I read your article, I felt like an expert in this field. I have several articles on these topics posted on my site. Could you please visit my homepage? Nhà cái lừa đảo
You can work as an intern and execute a live project for 2 months along with the course to make yourself a pro.
This was really an interesting topic and I kinda agree with what you have mentioned here! 토토사이트
This particular papers fabulous, and My spouse and i enjoy each of the perform that you have placed into this. I’m sure that you will be making a really useful place. I has been additionally pleased. Good perform! 먹튀검증
I’m going to read this. I’ll be sure to come back. thanks for sharing. and also This article gives the light in which we can observe the reality. this is very nice one and gives indepth information. thanks for this nice article... 구글상위노출
Average Andy Becomes a 토토검증업체 Dealer at Hard Rock Hotel
Greetings! Very helpful advice on this article! It is the little changes that make the biggest changes. Thanks a lot for sharing! Feel free to visit my website;
야설
Aw, this was a very good post. Taking the time and actual effort to create a good article… but what can I say… I put things off a whole lot and never seem to get nearly anything done Feel free to visit my website; 한국야동
You make so many great points here that I read your article a couple of times. Your views are in accordance with my own for the most part. This is great content for your readers. Feel free to visit my website; 국산야동
I was very pleased to find this page. I wanted to thank you for your time due to this wonderful read!! I definitely loved every part of it and I have you bookmarked to check out new information on your blog. Feel free to visit my website; 일본야동
Positive site, where did u come up with the information on this posting?I have read a few of the articles on your website now, and I really like your style. Thanks a million and please keep up the effective work. Feel free to visit my website; 일본야동
I wanted to thank you for this excellent read!! I definitely loved every little bit of it. I have you bookmarked your site to check out the new stuff you post. 안전놀이터
Greatly composed article, if just all bloggers offered a similar substance as you, the web would be a much better spot. bitmain antminer s19j pro
whats up, i do think that is a splendid net web page. I stumbledupon it 😉 i can revisit over again because of the fact that i ebook marked it. Cash and freedom is the greatest manner to change, can also you be wealthy and maintain to help others. It’s almost impossible to find skilled people approximately this topic, but, you sound like you know what you’re speaking approximately! Thanks . When i to start with left a comment i seem to have clicked the -notify me whilst new comments are delivered- checkbox and now each time a commentary is brought i recieve 4 emails with the exact equal observation. There ought to be a method you could put off me from that carrier? 토토안전센터
terrific look at, i simply exceeded this onto a colleague who became performing some have a take a look at on that. And he truely offered me lunch due to the fact i found it for him smile so allow me rephrase that: which can be in reality outstanding. Most of the people of tiny specs are unique owning lot of song record competence. I am just searching out to it over again quite masses. I found that is an informative and thrilling post so i assume so it's far very useful and informed. I would like to thanks for the efforts you have got got made in writing this article. I'm definitely getting a charge out of inspecting your flawlessly framed articles. In all likelihood you spend a considerable measure of exertion and time in your blog. I've bookmarked it and i'm anticipating investigating new articles. Hold doing astounding. i really like your positioned up. It's miles very informative thank you masses. In case you want cloth building . Thank you for giving me beneficial data. Please hold posting genuine data inside the destiny . Nice to be visiting your blog over again, it's been months for me. Well this article that ive been waited for consequently prolonged. I need this text to finish my task in the university, and it has identical subject matter together along with your article. Thanks, best proportion. I wanted to thanks for this to your liking ensnare!! I in particular enjoying all tiny little little bit of it i have you ever ever ever bookmarked to test out introduced belongings you pronounce. The blog and records is exceptional and informative as nicely . Superb .. Splendid .. I’ll bookmark your weblog and take the feeds also…i’m happy to locate such a lot of beneficial records here inside the post, we need exercise consultation more techniques on this regard, thank you for sharing. I need to say best that its amazing! The blog is informational and constantly produce notable things. Top notch article, it is in particular beneficial! I quietly began on this, and i'm turning into greater familiar with it better! Delights, preserve doing extra and additional brilliant 먹튀검증
this article is genuinely includes lot extra information approximately this topic. We've got observe your all the statistics a few factors are also specific and some usually are remarkable. Super post i would like to thank you for the efforts . Thank you for sharing this first-class stuff with us! Hold sharing! I am new within the blog writing. All sorts 토토사이트
tremendous information! I latterly got here throughout your blog and were reading along. I concept i might leave my first remark. I don’t realize what to mention except that i've. You may moreover check my weblog .! Positive i'm absolutely agreed with this article and i simply need say that this newsletter might be very exceptional and very informative article. I'm able to ensure to be reading your blog greater. You made an amazing point but i can't assist however wonder, what about the alternative factor? !!!!!! Thanks 토토사이트추천
Hi there friends, its great paragraph regarding teachingand completely defined, keep it up all the time. This is kind of off topic but I need some help from an established blog. Is it hard to set up your own blog? I’m not very techincal but I can figure things out pretty quick. I’m thinking about setting up my own but I’m not sure where to begin. Do you have any tips or suggestions? Thank you . Every weekend i used to visit this web site, because i wish for enjoyment, as this this web site conations truly nice funny material too. Have you ever thought about adding a little bit more than just your articles? I mean, what you say is important and everything. But imagine if you added some great pictures or video clips to give your posts more, “pop”! Your content is excellent but with pics and videos, this website could undeniably be one of the greatest in its niche. Fantastic blog! 먹튀패스
Hi there friends, its great paragraph regarding teachingand completely defined, keep it up all the time. This is kind of off topic but I need some help from an established blog. Is it hard to set up your own blog? I’m not very techincal but I can figure things out pretty quick. I’m thinking about setting up my own but I’m not sure where to begin. Do you have any tips or suggestions? Thank you . Every weekend i used to visit this web site, because i wish for enjoyment, as this this web site conations truly nice funny material too. Have you ever thought about adding a little bit more than just your articles? I mean, what you say is important and everything. But imagine if you added some great pictures or video clips to give your posts more, “pop”! Your content is excellent but with pics and videos, this website could undeniably be one of the greatest in its niche. Fantastic blog! 헤이먹튀
I'm not sure where you're getting your info, but great topic. I needs to spend some time learning more or understanding more. Thanks for magnificent info I was looking for this info for my mission. it's my first go to see at this web site, and post is really fruitful in favor of me, keep up posting such content. I am not naming which soft books I use, as I would not like it to be attainable for any soft book to identify me as the author of this short article. Sweet blog! I found it while browsing on Yahoo News. Do you have any tips on how to get listed in Yahoo News? I've been trying for a while but I never seem to get there! Thank you 무료가족방
hello. Cool post. There’s an trouble with your website in chrome, and you can want to test this… the browser is the market leader and a very good element of humans will omit your notable writing due to this problem. Very thrilling subject matter, thank you for posting. “the deepest american dream isn't always the starvation for cash or reputation it's far the dream of settling down, in peace and freedom and cooperation, inside the promised land.” with the aid of scott russell sanders.. Your internet site is clearly cool and that is a awesome inspiring article. 카이소
i’m inspired, i've to mention. Actually now not regularly must i encounter a blog that’s each educative and interesting, and really, you’ve hit the nail in the head. Your idea is terrific; the component is an trouble that too few folks are speakme intelligently approximately. I'm extremely joyful which i got here across this internal my discover some factor with this. Im impressed. I dont think ive met all of us who is aware of as a good deal about this case as you do. Youre really well knowledgeable and sincerely smart. You wrote some thing that people could apprehend and made the trouble exciting for every body. Certainly, notable weblog youve got here. It’s hard to gather informed human beings in this concern remember, nevertheless, you sound like what you are dealing with! Thanks 온카맨
I'm not sure where you're getting your info, but great topic. I needs to spend some time learning more or understanding more. Thanks for magnificent info I was looking for this info for my mission. it's my first go to see at this web site, and post is really fruitful in favor of me, keep up posting such content. I am not naming which soft books I use, as I would not like it to be attainable for any soft book to identify me as the author of this short article. Sweet blog! I found it while browsing on Yahoo News. Do you have any tips on how to get listed in Yahoo News? I've been trying for a while but I never seem to get there! Thank you 토토매거진
greetings! That is my first visit to your blog! We are a crew of volunteers and beginning a new initiative in a community inside the identical location of interest. Your blog supplied us beneficial facts to paintings on. You have completed a exquisite process! I wish i had discovered this weblog in advance than. The recommendation on this publish may be very beneficial and that i virtually will observe the alternative posts of this collection too. Thanks for posting this. You've got a superb website, well constructed and really interesting i have bookmarked you with any luck you hold posting new stuff. Hold up the top notch paintings , i study few content material on this net web page and i assume that your weblog may be very interesting and consists of devices of awesome facts . You introduced such an impressive piece to read, giving every venture enlightenment for us to gain records. Thank you for sharing such statistics with us because of which my numerous ideas were cleared. This is extraordinarily captivating substance! I have altogether overjoyed in perusing your focuses and characteristic arrived at the belief that you are exceptional approximately a massive variety of them. You are superb. I am grateful to you for sharing this plethora of useful facts. I found this resource utmost beneficial for me. Thanks masses for difficult artwork. I absolutely like evaluate dreams which understand the value of passing at the first rate strong asset futile out of pocket. I surely worshiped reading your posting. Thankful to you! 안전토토사이트
Thanks for sharing this blog its very helpful to implement in our work
French Classes in Chennai | French Institute in Chennai
To know about automated help desk and service desk with rezolve.ai click here: https://www.rezolve.ai/home
Wow, amazing blog layout! How long have you been blogging for? you make blogging look easy. 토토먹튀
You made some good points there. I did a Google search about the topic and found most people will believe your blog 안전토토사이트
Thank you very much for sharing this article. It helped me a lot and made me feel a lot. Please feel free to share such good 토토검증업체
I was looking for a site that posted these useful information and I think I found it now. Thank you. 안전토토
I ve recently been thinking the very same factor personally lately. Delighted to see a person on the same wavelength! Nice 토토먹튀검증
As expected, I can only see articles that can grow. I ll let you know around me. 토토사이트
Good post. I learn something new and challenging on websites.
Mumbai Vip Girls |
Bhabhi in Andheri ||
Bandra Hot Girls ||
Independent Girls in Borivali ||
Chembur College Girls ||
Airhostess Girls in Colaba ||
Introducing a new application named Engadoctor. Especially design with newly emerging technology for Online Doctor Consultation & Book Online Doctor Appointment.
CasinoMecca
Excellent Blog! I would like to thank for the efforts you have made in writing this post. I am hoping the same best work from you in the future as well. I wanted to thank you for this websites! Thanks for sharing. Great websites! This article gives the light in which we can observe the reality. This is very nice one and gives indepth information. Thanks for this nice article. I would also motivate just about every person to save this web page for any favorite assistance to assist posted the appearance. Nice post. I was checking continuously this blog and I am impressed! Extremely useful info particularly the last part :) I care for such information much. I was seeking this particular info for a long time. Thank you and good luck. 오래된토토사이트
Thank you of this blog. That’s all I’m able to say. You definitely have made this web site into an item thats attention opening in addition to important. You definitely know a great deal of about the niche, youve covered a multitude of bases. Great stuff from this the main internet. All over again, thank you for the blog. This unique looks utterly perfect. Every one of plain and simple records are prepared through the help of great number for working experience handy experience. I will be happy it all for a second time considerably. Thank you so much for such a well-written article. It’s full of insightful information. Your point of view is the best among many without fail.For certain, It is one of the best blogs in my opinion. 안전놀이터홍보
Hi, i think that i saw you visited my site so i came to “return the favor”.I am attempting to find things to enhance my website!I suppose its ok to use a few of your ideas!! I’m really glad I’ve found this information. Nowadays bloggers publish only about gossips and internet and this is actually irritating. A good blog with exciting content, this is what I need. Thanks for keeping this web-site, I will be visiting it. Thanks a lot for giving everyone remarkably marvellous chance to check tips from here. It can be very pleasant and as well , stuffed with a great time for me and my office friends to visit the blog 토토사이트
Thank you for this wonderful post! It has long been extremely helpful. 메이저사이트I wish that you will carry on posting your knowledge with us.
I set up a bookmark and log on every time. Good. I m leaving a comment today as well. 먹튀폴리스
Your writing is perfect and complete. majorsite However, I think it will be more wonderful if your post includes additional topics that I am thinking of. I have a lot of posts on my site similar to your topic. Would you like to visit once?
It's really great. Thank you for providing a quality article. There is something you might be interested in. Do you know casinosite ? If you have more questions, please come to my site and check it out!
Please keep on posting such quality articles as this is a rare thing to find these days. I am always searching online for posts that can help me. watching forward to another great blog. Good luck to the author! all the best! 스포츠토토사이트
XDG
It's the same topic , but I was quite surprised to see the opinions I didn't think of. My blog also has articles on these topics, so I look forward to your visit. 카지노사이트추천
This very informative and interesting blog. I have read many blog in days but your writing style is very unique and understanding. if you are interested in home appliances then click below.
buy home appliances
buy home appliances online
Nice post. Thanks for sharing! I want people to know just how good this information is in your article. It’s interesting content and Great work.
Youtube training course in hyderabad
"Trying to say thank you won't simply 파워볼
Pretty nice post. I just stuem. It seems to me all of them are brilliant. 토토경비대
allrecipes-1
Allrecipes-1 is a collection of recipes that offers a wide range of dishes for people to try. It includes recipes for appetizers, main courses, desserts, and more. The website allows users to search for specific recipes or browse through different categories. Allrecipes-1 is a valuable resource for anyone looking to explore new recipes and expand their cooking skills.
allrecipes-1
allrecipes-1
Allrecipes-1 is a collection of recipes that offers a wide range of dishes for people to try. It includes recipes for appetizers, main courses, desserts, and more. The website allows users to search for specific recipes or browse through different categories. Allrecipes-1 is a valuable resource for anyone looking to explore new recipes and expand their cooking skills.
https://allrecipes-1.blogspot.com
Need help to afford expensive streaming services? Learn how Pikashow gives you unlimited access to movies and shows without any subscription fees. This post covers everything you need to know about this free streaming site.
pikashow
Stop wasting money on expensive streaming subscriptions. This post introduces Pikashow, the 100% free streaming site that makes watching movies and TV affordable again.
pikashow
Tired of ads on YouTube? Learn how to install YouTube Vanced, an ad-free mod of the YouTube app for Android. We cover the download, features, and step-by-step installation guide.
https://youtubevanced.com.in
Looking for the best deals on your favorite brands? The innovative temu App leverages the power of bulk buying to offer prices you won't find anywhere else. Our review explores whether it's too good to be true.
Can an app really offer brand-name products at 90% off retail prices? Our review of the buzzworthy temu App investigates whether its rock-bottom prices are the real deal or too good to be true.
Give your Android device the ultimate musical upgrade with the spotify premium apk. This post has everything you need to know to get premium features for free.
minecraft apk brings the endless creative possibilities of the PC game to mobile. Download it now and start building imaginative worlds on your phone.
Experience the creative magic of minecraft apk on your phone or tablet. Get the APK to start playing one of the most popular sandbox games ever made.
Can't get enough of your favorite Korean and Japanese TV shows? doramasflix
has you covered with the largest collection of Asian dramas online. Learn more about this top streaming site for doramas.
doramasflix
is every K-drama and J-drama fan's dream streaming site. With thousands of episodes subbed and readily available, you'll never run out of shows to watch. Our review has all the details.
If you love streaming the hottest new Asian dramas, you need doramasflix in your life. This post explores the user-friendly interface, extensive media library and awesome features that make Doramasflix a must-use free streaming site.
estrenos doramas 2023. Descubre las nuevas y emocionantes series coreanas que llegan este año. Romance, thriller y comedia en estos esperados estrenos que cautivarán a los fans del k-drama.
Llegan nuevos estrenos doramas que te engancharán desde el primer capítulo. Las mejores series coreanas con actores de renombre y tramas adictivas. No te pierdas los estrenos doramas de este año.
From drama to romance, desi serials have something for everyone. This post dives into the top Hindi TV shows and why generations of viewers can't get enough. Learn what makes these serials so binge-worthy.
Addicted to desi serials? You're not alone. This blog looks at why these Hindi TV shows are so popular, from the relatable characters to the slow-burn storytelling style that keeps viewers hooked.
Want to create awesome videos to share online without fancy software? The capcut app makes video editing incredibly simple. This post explains how to use Capcut.
Tired of expensive cable bills just to watch desi TV? We review affordable desi tv box options that provide live streaming access to all your favorite South Asian channels.
Get your daily dose of drama and entertainment with this in-depth blog exploring the most popular Hindi serials airing on leading channel star plus serials
. From storylines to characters to future episodes - find everything you want to know about Star Plus serials here.
estrenos doramas: fecha, reparto, trama y tráiler de las nuevas series coreanas y japonesas que se estrenarán próximamente. Te contamos todos los detalles.
Immerse yourself in the world of Korean dramas with doramaswow, your one-stop shop for streaming and discussing beloved K-dramas. From trending rom-coms to critically acclaimed series, satisfy your Hallyu cravings at
doramaswow
Say goodbye to video ads with youtube vanced mod apk This handy modded YouTube app blocks all ads so you can enjoy videos without interruptions. Get it now!
faceapp mod apk Experience this viral selfie editor with all features unlocked - no ads, no payments. Download the FaceApp mod and get unlimited use of filters like age, smile, and more.
Unlock the full potential of lightroom mod apk latest version 2024 with this 2024 mod apk. The latest version has all premium features for free - presets, advanced editing tools and more.
Get ready to feel nostalgic! This guide will show you how to download the oludo king old version and play the classic game we enjoyed before all the updates.
Tired of limited edits? Upgrade to the new picsart mod apk latestfor unlimited layers, fonts, tools and more, all without ads. Download this mod and take your photo editing to the next level.
If you want to watch movies and shows for free on your Android, the pikashow apk is one of the top options. Learn all about this media player app and how to get it on your device.
Turn back time and download an old mod of the spotify mod apk old version. This classic APK unlocks premium benefits like no ads and unlimited skips for free.
Join us on a musical journey through time as we unpack spotify mod apk old versionK origins. This post offers a fascinating look back at early hacked and modded APK versions of Spotify through the years.
Get nostalgic with a blast from the past! This guide has everything you need to download and install an old version of the highly popular spotify mod apk old version
on your Android device.
Tired of YouTube ads? youtube vanced manager offers the solution you need. Read this post to learn how it gives you greater control over your YouTube experience and removes all those annoying ads.
Los fans del K-drama tienen mucho que esperar este año. Descubre los nuevos estrenos doramas coreanos más emocionantes con esta lista que detalla las próximas series que no te puedes perder.
Πραγματικά κατατοπιστικό άρθρο post.Really ανυπομονούμε να διαβάσετε περισσότερα. Θέλω περισσότερα.
Training Medal
I feel strongly about this and love learning a great deal more on this subject.
Thank you for posting; I understood what you meant.
Wow, I'm glad I came into your website via Google.
Post a Comment